|
MAIN PAGE
> Back to contents
Security Issues
Reference:
Mishin A., Andriyanov N.A.
Indicators for assessing the risk of execution of state contracts with a long life cycle
// Security Issues.
2023. ¹ 4.
P. 39-59.
DOI: 10.25136/2409-7543.2023.4.44047 EDN: AIIJFD URL: https://en.nbpublish.com/library_read_article.php?id=44047
Indicators for assessing the risk of execution of state contracts with a long life cycle
Mishin Aleksandr
ORCID: 0000-0002-0592-8524
PhD in Economics
Associate Professor of the Department of Business Informatics, Faculty of Information Technologies and Big Data Analysis, Financial University under the Government of the Russian Federation
125167, Russia, Moscow, 49/2 Leningradsky Ave.
|
mishin_au@mail.ru
|
|
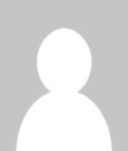 |
|
Andriyanov Nikita Andreevich
ORCID: 0000-0003-0735-7697
PhD in Technical Science
Associate Professor, Department of Data Analysis and Machine Learning Faculty of Information Technologies and Big Data Analysis, Financial University under the Government of the Russian Federation
125167, Russia, Moscow, 49/2 Leningradsky Ave.
|
naandriyanov@fa.ru
|
|
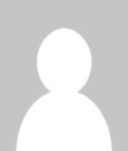 |
|
DOI: 10.25136/2409-7543.2023.4.44047
EDN: AIIJFD
Received:
16-09-2023
Published:
16-11-2023
Abstract:
The subject of the study is the process of monitoring the execution and assessing the risk of execution of government contracts in the Russian Federation. The research methodology consists of studying statistical data on government procurement in Russia; enriching data from the UIS with additional data from market analysis systems; analyzing the subject area and identifying potentially valuable categorical data that have not been previously studied by other scientists and applying the tools of one-way analysis of variance to these data in order to assess their statistical significance for solving problems of predicting the execution of government contracts. By applying the ANOVA method to a dataset that included more than 83 thousand consolidated records, results were obtained confirming the significance of a number of categorical features relating to the supplier’s industry, its legal form and region for predicting the execution of government contracts. At the same time, it was revealed that such characteristics as form of ownership, method of placing an order, legal category of the size of the supplier’s business and budget level are not statistically significant for forecasting purposes. The results obtained can be used by researchers in the course of cluster analysis, exploratory data analysis, and when constructing an ensemble of models for predicting the execution of government contracts. The results obtained expand and deepen existing approaches in terms of searching for new significant features based on information capabilities contained in government information systems and other big data sources.
Keywords:
Government procurement, monitoring of contract execution, 44-FZ, unified information system in the field of public procurement, risk assessment, contractor, register of unscrupulous suppliers, model features, supplier assessment, financial indicators
This article is automatically translated.
You can find original text of the article here.
The article was prepared based on the results of research carried out at the expense of budgetary funds under the state assignment of the Financial University Introduction The chosen direction of research, firstly, indirectly affects all the national goals established by Decree of the President of the Russian Federation No. 474 of 21.07.2020, since the efficiency of the Russian economy is largely determined by the efficiency of its public sector due to its high share in it; secondly, it directly supports the goal "d", which is to "achieve digital maturity of key sectors of the economy and social sphere, including healthcare and education, as well as public administration." This study is relevant, since the volume of public procurement is comparable to the revenue part of the federal budget. So, in 2022, the volume of public procurement exceeded the volume of 12.9 trillion. rubles according to 44-FZ (Galieva D. Small tender, yes expensive. The newspaper "Kommersant" No. 1 of 09.01.2023, p. 2) and 8.1 trillion. 223-FZ. (The volume of purchases in the B2B segment in 2022 decreased in the Russian Federation by 2% to 33 trillion rubles. The official website of the Interfax agency). Within the framework of the existing public procurement system in Russia, there are a number of problems of the effectiveness of procurement processes. Yudin Yu.A., Tarabukina T.V., Oblizov A.V. (2023) argue that "during the execution of the contract, all savings of public funds can be spent on finalizing or correcting violations under the contract" [1]. It is also impossible not to agree with Melnikov V.V. (2022) that "the legislation on the contract system does not provide for the expert community and customers a common understanding of what "efficiency of public procurement" is and what are the criteria for achieving it" [2, p. 127] and "there has been an ideological departure from understanding the importance of the effectiveness of ensuring the needs of achieving budget savings while creating equal access to suppliers and transparency of transactions" [ibid., p.125]. It should be noted that this situation is observed even 16 years after the commissioning of the EIS and the accumulation of a huge amount of digital data. Thus, the share of failed and canceled contracts in the total number of notifications for 2022 amounted to 33.4% by the end of 2022, and more than half of this figure falls on transactions for which contracts were still concluded – 20.5% of the total number of notifications (calculated on the basis of data from the Consolidated Analytical Report on the results of monitoring purchases of goods, works, services for state and municipal needs, as well as purchases of goods, works, services by certain types of legal entities for 2022, developed by the Ministry of Finance of the Russian Federation, pp.45-46). Although the problems of the public procurement system in Russia are complex, the obvious point of applying efforts to optimize public procurement processes is the creation of a data-driven functionality built into the EIS for selecting suppliers and assessing the risks of executing government contracts. According to the research results of Styrin E. M., Rodionova Yu. D. (2020) "There is no predictive analytics system. On electronic platforms, only individual elements of proactive service provision can be identified" [3, p.61]. The existing functionality of the EIS allows the customer to set a system of criteria for selecting a supplier in manual mode, partially relying on the current legislative and regulatory requirements. At the same time, the supplier within the framework of the EIS still does not have access to raw data that allows profiling the supplier, and to recommendations based on big data analysis. Regulators – the Federal Treasury, the Federal Antimonopoly Service, the Ministry of Finance, the Accounting Chamber and other state authorities - are engaged in the analysis of these data. The result of such an analysis is regularly revised standards that are fixed in legislation and mandatory for all participants in public procurement (unless otherwise provided by law) (see Figure 1).
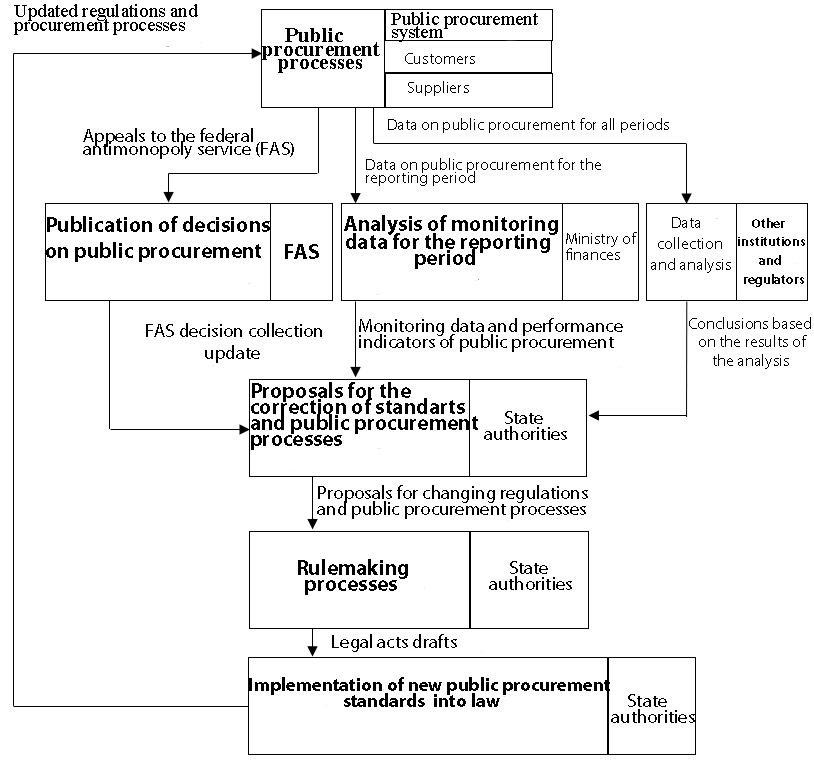
Figure 1. An enlarged scheme of the process of regulating the public procurement system in Russia Source: developed by the authors The disadvantages of the process model presented above are: 1) The duration and cost of making changes. The change of standards occurs through the introduction of amendments to regulatory legal acts; 2) The standards of the public procurement system are calculated only on the basis of indicators located inside the EIS circuit, and not on the basis of big data available to government agencies; 3) Data analysis and machine learning (ML) technologies are not used enough. It is possible to significantly improve the efficiency of public procurement processes by automating the procedure for evaluating and selecting a supplier on the basis of recommendation ML technologies, or by adding the functionality of forecasting contract performance with certain parameters to the EIS. For the development of such models, the key task will be to identify significant features that allow predicting the effectiveness of the concluded state contract. The object of the study is the processes of monitoring the execution and risk assessment of the execution of government contracts, and the subject of the study is the indicators of risk assessment of the execution of government contracts in the Russian Federation. The purpose of the study is to identify indicators (signs) of government contracts that may be important for predicting their execution. Review of existing research The analysis of literary sources in this area has shown that these problems have been attracting the attention of Russian and foreign scientists for quite a long time. In Western countries, there is no such regulated system of public procurement and the level of corruption may be less, the culture of contract fulfillment and fulfillment of obligations is significantly different, so there are no comprehensive studies in the perspective of the task of assessing the risks of already launched contracts. The most complete, though outdated study was conducted by Farzad Khosrowshahi (1999) [4]. In his work, many signs have been investigated, but it is entirely devoted to the analysis of the supplier exclusively, and not the contract or the signs of the procurement process. And this work came out at a time when there was still no big historical data. Its results are only partially applicable to Russian practice, as well as the work of Acheamfour Karikari V., Kissi E., Adjei-Kumi T., Adinyira E. (2020) [5]. Both works appeal to the features for which to automate the collection and calculation of data, for example, the reputation of the company, the experience of the staff, etc. El-Sawalhi N., Eaton D., Rustom R. (2007) also agree with these authors [6]. Eliseev D., Dmitry Romanov D. (2018) have created a fairly effective assessment forecasting model that includes only four key criteria and is well suited for Russian conditions [7]. Sozaeva D.A., Gonchar K.V. (2022) used BI-solutions for visual data analysis, and did not evaluate the statistical significance of features for the purposes of forecasting the execution of government contracts, indicating the importance of OKPD 2 as a key feature affecting the result of contract execution [8]. The authors also considered any early termination of the contract as a target feature, while in the framework of this study, the authors decided to consider the termination of the contract by agreement of the parties as a successful completion of the contract, since there were a lot of such cancellations after 2022, and they are associated with difficulties experienced not only by suppliers, but also by customers. The works of Sanjana P., Basavaraj K., Pranali D, Kashmira P. (2020) [9] and Zedan A. (1996) [10] do not contain fundamental additions to the aforementioned authors, highlighting the importance of construction projects as the most problematic in terms of performance efficiency. Table 1 presents the main studies in this area, containing a list of the most significant features of contracts for predicting their execution. Table 1. Overview of existing research in the field of identifying key features for predicting the effectiveness of the execution of government contracts No. p / p | Authors | Key features | 1 | Farzad Khosrowshahi (1999) | - financial position and portfolio;
- general experience;
- reputation for performing work on time;
- reputation for high quality service;
- attitude to occupational health and safety;
- post-business relationship;
- project cost;
- effective organization;
- staff/team experience;
- recent experience in similar projects;
- level of technical equipment [4]
| 2 | Acheamfour Karikari V., Kissi E., Adjei-Kumi T., Adinyira E. (2020) | - staff experience;
- financial stability;
- number of years in the industry;
- the quality level of contract execution;
- past customer relationships;
- technical equipment;
- past failures in completed contracts;
- credit rating;
- violation of deadlines for the execution of past contracts;
- availability of a quality control system [5]
| 3 | Eliseev D., Dmitry Romanov D. (2018) | - supplier's experience;
- type of the customer's organization;
- average contract price;
- experience in executing specialized contracts [7]
| 4 | D.A. Sozaeva, K.V. Gonchar (2022) | - OKPD2 code
- number of participants per purchase procedure;
- grounds for termination of the contract [8]
| Source: developed by the authors Tsyganova M. S., Chernushenko D. A., Buresh S. V. (2022) revealed the influence of the seasonal factor on procurement efficiency, although they did not directly address forecasting issues in their work [11]. In this study, when uploading a data set, this is taken into account by forced random sampling for various years and months due to restrictions imposed by the peculiarities of the operation of the EIS server at this time.
Research methodology As part of the study, the authors applied the approaches of Exploratory Data Analysis (EDA). Schematically, the methodology of the study is presented in Figure 2.
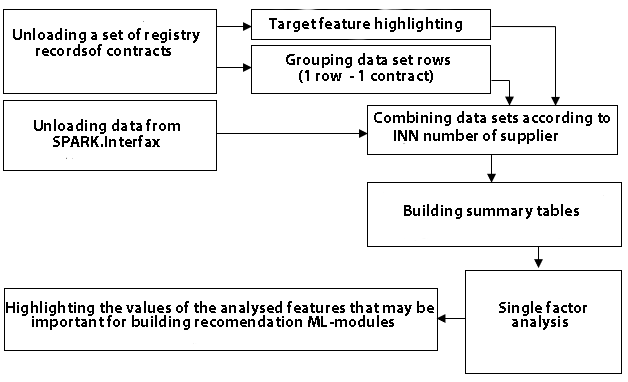
Figure 2. Stages of the study Source: developed by the authors Data was uploaded using the standard EIS upload service. This service has a number of restrictions regarding the number of contracts to be uploaded per operation and the absence of a number of features in the uploaded data set (if they are present in the tools of the contract search and filtering interface). Data was uploaded by repeating similar requests to the EIS for different years and combining the received uploads into one data set with the addition of a feature not displayed in the upload manually. In addition to all twenty-nine signs available in the EIS for unloading contracts, the signs "Status" and "Reason for termination" were manually added to the data set, having the following values: Values of the "Status" attribute: "Execution completed", "Execution terminated". The values of the attribute "Reason for termination": "No", "Unilateral refusal of the customer from the performance of the contract in accordance with civil law", "Unilateral refusal of the supplier (contractor, contractor) from the performance of the contract in accordance with civil law", "Agreement of the parties", "Judicial act". Next, a target attribute was added to the data set, reflecting the presence or absence of problems with the execution of the state contract. The target attribute was assigned the value "1" if the attribute "Status" has the value "Performance terminated" and the attribute "Reason for termination" has one of the following meanings: "Unilateral refusal of the customer from the performance of the contract in accordance with civil law", "Unilateral refusal of the supplier (contractor, contractor) from the performance of the contract in accordance with in accordance with civil law", "Judicial act". Accordingly, if the contract is completed normally, then the "Status" sign has the value "Execution completed". Also, the contract within the framework of the study is considered successfully executed if the "Status" sign has the value "Performance terminated", but the reason for termination is not specified or has the value "Agreement of the parties". At this stage, the number of records in the dataset was more than 1 million. The unique identifier of the contract is the "Registry Entry number" field, and some registry entries with about thirty thousand rows were identified in the data set. Lines with the same numbers of registry entries differ in quantity, price, cost, identifiers of products supplied / services rendered (in fact, according to the lines of the contract specification). With the help of one VBA procedure, unique values of contract registry records were found and unloaded, and with the help of another, derived signs were added to the data set: "There were rows in the registry record", "Total units of products / services actually delivered", "Total cost of delivered products". The addition of these features made it possible to compress the data set to about 83 thousand records without losing important information about contracts. The choice of VBA as a data processing tool was due to the large amount of data. The codes of the developed procedures are shown in Figures 3 and 4.
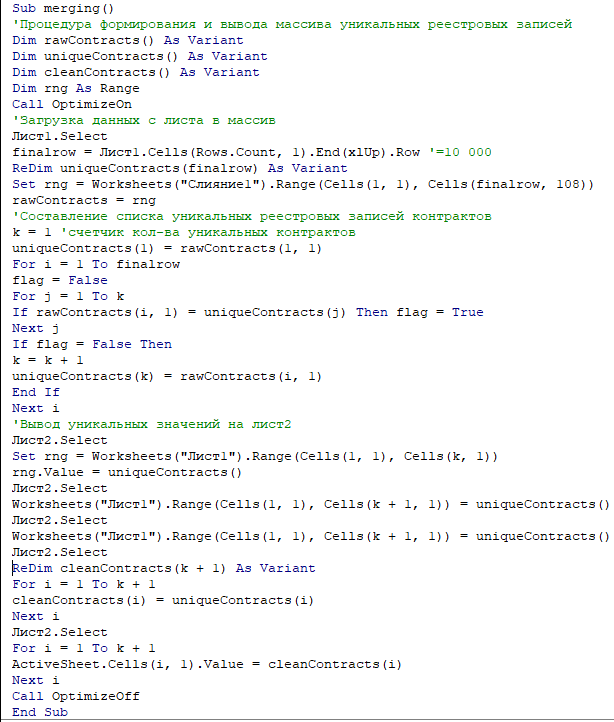
Figure 3. VBA code-procedures for finding unique numbers of registry entries Source: developed by the authors
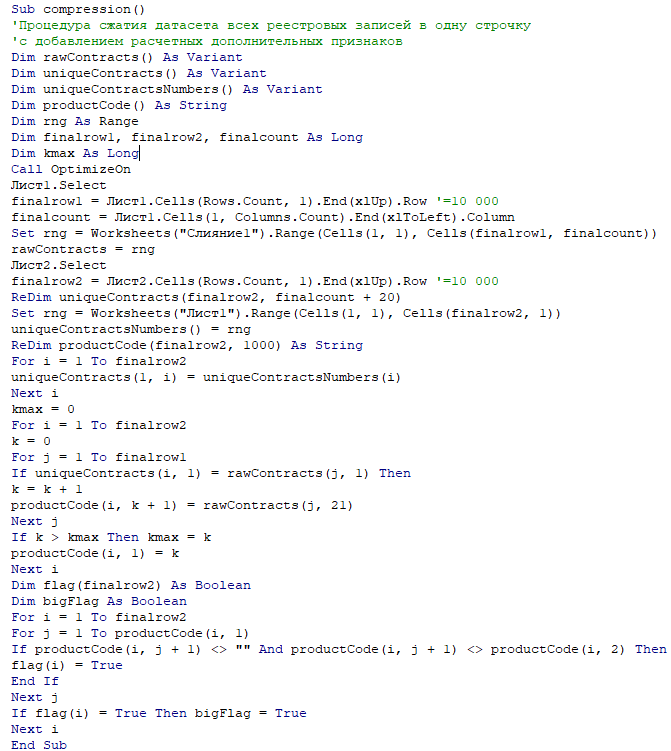
Figure 4. VBA code-procedures for compressing a data set by unique numbers of registry entries Source: developed by the authors In parallel with the data processing by standard services, a data set for supplier companies was unloaded from the Spark/Interfax service in order to enrich the original dataset. To do this, at first, based on the data of a compact source dataset, MS Excel tools found unique TIN suppliers. The generated csv file was used as input data for uploading a data set from the above service. The resulting data set enriched the original dataset with 135 features. Due to the large number of signs, their full list is not given in the study. The structure of the added features is presented in Table 2. Table 2. Overview of existing research in the field of identifying key features for predicting the effectiveness of the execution of government contracts No. p / p | Feature group | Number of signs in the group | 1 | Financial and economic indicators for 2021 and 2022 | 101 | 2 | Legal signs | 30 | 3 | Signs that do not contain valuable information (duplicate identifiers, notes, text signs with unstructured information and low power, etc.) | 4 | Source: developed by the authors Since the financial and economic indicators of suppliers have already been well studied by previous authors (their work has already been reviewed above), and the purpose of the study is to increase scientific knowledge, in order to find new valuable features, the authors focused on analyzing the significance of categorical features that, according to the results of the domain analysis, can be useful in assessing the risks of the contract, and in at the same time, they were not previously considered by other authors. In total, within the framework of the consolidated data set, the authors selected the following list of features for further analysis: - Form of ownership;
- Organizational and legal form;
- Company Size;
- Budget level;
- Supplier's region;
- The first two characters of the OKVED 2 code;
- The method of placing the order.
Further, conjugation tables (summary tables) were constructed for the selected categorical features. Its example for the attribute "Form of ownership" is presented in Table 3: Table 3. The conjugation table for the attribute "Form of ownership" No. p / p | The meaning of the attribute "Form of ownership" | Number of successful contracts | Number of unsuccessful contracts | total | 1 | A different mix. Ross. | 28 | 522 | 550 | 2 | Municip. | 100 | 4214 | 4314 | 3 | Mixed. inostr. | 1 | 0 | 1 | 4 | Mixed. Ross. with the share of the subjects of the Russian Federation | 20 | 739 | 759 | 5 | Mixed. Ross. with a share of fed. | 18 | 2069 | 2087 | 6 | Mixed. Ross. with shares fed. and subjects of the Russian Federation | 21 | 95 | 116 | 7 | Blagotv. org. | 1 | 6 | 7 | 8 | State Corp. | 2 | 247 | 249 | 9 | In. civil. and persons without gr. |
101 | 151 | 252 | 10 | In. YUL | 14 | 145 | 159 | 11 | Inter. org. | 0 | 1 | 1 | 12 | General. obed. | 42 | 257 | 299 | 13 | Consumer. co-op. | 0 | 45 | 45 | 14 | Trade unions | 2 | 44 | 46 | 15 | Subjects of the Russian Federation | 47 | 4306 | 4353 | 16 | Council of Subjects of the Russian Federation and foreign | 0 | 93 | 93 | 17 | Sovm. fed. and foreign | 1 | 18 | 19 | 18 | Sovm. private and foreign. | 39 | 707 | 746 | 19 | Federal | 32 | 1372 | 1404 | 20 | Private | 10768 | 57558 | 68326 | | total | 11237 | 72589 | 83826 | Source: developed by the authors Attention is drawn to the large spread of the values of the target attribute across the selected groups of the studied attribute. For the obtained data, the use of Pearson's chi-squared criterion is inappropriate, since the obtained values of the criterion will be unrealistic due to the presence of zeros in the sample and uneven frequencies. In this case, it is more correct to use the exact Fischer test, however, this method is poorly optimized for conjugation tables of the dimension required in this study. The need to calculate large factorials taking into account huge frequencies quickly causes a RAM overflow error. The problem will be solved in the framework of further research by the authors by developing a special numerical algorithm. Since the purpose of this work is to find a list of new significant features that could be used in forecasting the execution of state contracts, it was decided to use one-factor analysis of variance (ANOVA). Tables 4 and 5 show its results for the data given earlier in Table 3. Table 4. Calculation of averages and variances for groups of the attribute "Form of ownership" No. p / p | Groups | Account | The amount | Average | Variance | 1 | A different mix. Ross. | 2 | 550 | 275 | 122018 | 2 | Municip. | 2 | 4314 | 2157 | 8462498 | 3 | Mixed. inostr. | 2 | 2 |
1 | 0 | 4 | Mixed. Ross. with the share of the subjects of the Russian Federation | 2 | 759 | 379,5 | 258480,5 | 5 | Mixed. Ross. with a share of fed. | 2 | 2087 | 1043,5 | 2103300,5 | 6 | Mixed. Ross. with shares fed. and subjects of the Russian Federation | 2 | 116 | 58 | 2738 | 7 | Blagotv. org. | 2 | 7 | 3,5 | 12,5 | 8 | State Corp. | 2 | 249 | 124,5 | 30012,5 | 9 | In. civil. and persons without gr. | 2 | 252 | 126 | 1250 | 10 | In. YUL | 2 | 159 | 79,5 | 8580,5 | 11 | Inter. org. | 2 | 2 | 1 | 0 | 12 | General. obed. | 2 | 299 | 149,5 | 23112,5 | 13 | Consumer. co-op. | 2 | 45 | 22,5 | 1012,5 | 14 | Trade unions | 2 | 46 | 23 | 882 | 15 | Subjects of the Russian Federation | 2 | 4353 | 2176,5 | 9069540,5 | 16 | Council of Subjects of the Russian Federation and foreign | 2 | 93 | 46,5 | 4140,5 | 17 | Sovm. fed. and foreign | 2 | 19 | 9,5 | 144,5 | 18 | Sovm. private and foreign. | 2 | 746 | 373 | 223112 | 19 | Federal | 2 | 1404 | 702 | 897800 | 20 | Private | 2 | 68326 | 34163 | 1094652050 | Source: developed by the authors Table 5. Results of one-factor analysis of variance of the attribute "Form of ownership" No. p / p | Source of variation | SS | df | MS | F | P-Value | F critical | 1 | Between groups | 2181336767 | 19 | 114807198,3 | 2,057733547 | 0,058832576 | 2,137008959 | 2 | Within groups |
1115860685 | 20 | 55793034,25 | | | | 3 | Total | 3297197452 | 39 | | | | | Source: developed by the authors Based on the results of the calculation of ANOVA indicators, it can already be concluded at this stage that this feature does not exactly have significance and is not valuable for predicting the execution of contracts, since the P-value is greater than 0.05. Further, according to the described methodology, the ANOVA method will be applied to the remaining selected features. Research results and their discussion The author suggests using a number of indicators developed on the basis of data available in state information systems to assess the risk level of execution of a specific contract. Table 6 shows the results of applying the ANOVA method to the feature "The first two characters of the OKVED 2 code". Table 6. Results of one-factor analysis of variance of the feature "The first two characters of the OKVED 2 code" No. p / p | Source of variation | SS | df | MS | F | P-Value | F critical | 1 | Between groups | 716143328 | 83 | 8628232,87 | 1,497817641 | 0,033229134 | 1,43597929 | 2 | Within groups | 483885048 | 84 | 5760536,29 | | | | 3 | Total | 1200028376 | 167 | | | | | Source: developed by the authors Thus, within the framework of the study, it was revealed that the industry affiliation of the supplier organization is important for predicting the execution of a government contract (the calculated P-value is less than 0.05). The tables of conjugation and calculation of averages and variances are not given, because the number of unique high-order values of OKVED 2 included in the sample was 84. The same decision was made with respect to the "Supplier region" attribute (see table 7): Table 7. Results of one-factor analysis of variance of the "Supplier region" attribute No. p / p | Source of variation | SS | df | MS | F | P-Value | F critical | 1 | Between groups | 136503013,7 | 85 | 1605917,808 | 1,576396844 | 0,018286662 | 1,429821618 | 2 | Within groups | 87610510 | 86 | 1018726,86 | | | | 3 | Total | 224113523,7 | 171 | |
| | | Source: developed by the authors This feature may also be statistically significant for predicting the target feature. Consider the "Order placement method" attribute (Table 8). Table 8. Interface table for the "Order placement method" attribute No. p / p | The value of the "Order placement method" attribute | Number of successful contracts | Number of unsuccessful contracts | total | 1 | Zak. u ed. post. for the amount according to Part 12 of Article 93 44-FZ | 0 | 94 | 94 | 2 | Zak. u ed. post. | 686 | 22549 | 23235 | 3 | Cat request in the email form | 447 | 3081 | 3528 | 4 | The request is submitted. in electronic form | 13 | 24 | 37 | 5 | Competition with limited participation in electronic form | 61 | 1738 | 1799 | 6 | Competition with limited participation (science, cult, art) | 0 | 1 | 1 | 7 | Open the contest in electronic form | 728 | 3194 | 3922 | 8 | Open the competition in the electronic form for the audit of the audit (part 4 of Article 5 307-FZ) | 1 | 5 | 6 | 9 | Open competition in electronic form (science, cult, art) | 0 | 2 | 2 | 10 | Open competition in electronic form (energoservice) | 0 | 5 | 5 | 11 | Open the competition in electronic form (page, reconstruction, cap. rem., demolition of the object cap. p. according to part 1 of paragraph 8 of Article 33 44-FZ) | 53 | 84 | 137 | 12 | Open the competition in electronic form (page, reconstruction, cap. rem., demolition of the object cap. p., provided. projectn. dokum. repeat. use. … | 81 | 110 | 191 | 13 | Ust. Pr-vom RF according to art. 111 44-FZ | 3 | 41 | 44 | 14 | E-auction | 8465 | 40928 | 49393 | 15 | Electronic auction (energy service) | 0 | 1 | 1 | 16 | Electronic auction (solid. comm. waste) | 0 | 1 | 1 | 17 | Electronic auction (page, reconstruction, cap. rem., demolition of the object cap. p. according to part 1 of paragraph 8 of Article 33 44-FZ) | 347 | 446 | 793 | 18 | Electronic auction (page, reconstruction, cap. rem., demolition of the object cap. p., provided. projectn. dokum. repeat. use ...) | 360 | 285 | 645 | 19 | Total | 11245 | 72589 | 83834 |
Source: developed by the authors Tables 9 and 10 show its results for the data given earlier in Table 3. Table 9. Calculation of averages and variances for groups of the "Order placement method" attribute No. p / p | Account | The amount | Average | Variance | 1 | 2 | 94 | 47 | 4418 | 2 | 2 | 23235 | 11617,5 | 238995384,5 | 3 | 2 | 3528 | 1764 | 3468978 | 4 | 2 | 37 | 18,5 | 60,5 | 5 | 2 | 1799 | 899,5 | 1406164,5 | 6 | 2 | 1 | 0,5 | 0,5 | 7 | 2 | 3922 | 1961 | 3040578 | 8 | 2 | 6 | 3 | 8 | 9 | 2 | 2 | 1 | 2 | 10 | 2 | 5 | 2,5 | 12,5 | 11 | 2 | 137 | 68,5 | 480,5 | 12 | 2 | 191 | 95,5 | 420,5 | 13 | 2 | 44 | 22 | 722 | 14 | 2 | 49393 | 24696,5 | 526923184,5 | 15 | 2 | 1 | 0,5 | 0,5 | 16 | 2 | 1 | 0,5 | 0,5 | 17 | 2 | 793 | 396,5 | 4900,5 | 18 | 2 | 645 | 322,5 | 2812,5 | Source: developed by the authors Table 10. Results of one-factor variance analysis of the "Order placement method" attribute No. p / p | Source of variation | SS | df | MS | F | P-Value | F critical | 1 | Between groups | 1310629539 | 17 | 77095855 | 1,793278738 | 0,114414929 | 2,23254567 | 2 | Within groups | 773848128 | 18 | 42991563 | | | | 3 | Total | 2084477667 | 35 | | | | | Source: developed by the authors
Based on the results of the calculation of ANOVA indicators, it can be concluded that this feature is not significant and is not valuable for predicting the execution of contracts, since it has a P-value of 0.1144. Next, we will analyze the "Company size" attribute (see Table 11). Table 11. Interface table for the "Company size" attribute No. p / p | The value of the "Company size" attribute | Number of successful contracts | Number of unsuccessful contracts | total | 1 | Large enterprises | 305 | 19239 | 19544 | 2 | Small businesses | 1841 | 18443 | 20284 | 3 | Microenterprises | 7678 | 24619 | 32297 | 4 | Medium-sized enterprises | 208 | 5800 | 6008 | 5 | total | 10032 | 68101 | 78133 | Source: developed by the authors Tables 12 and 13 show its results for the data given in table 11. Table 12. Calculation of averages and variances for groups of the "Company size" attribute No. p / p | Groups | Account | The amount | Average | Variance | 1 | Large enterprises | 2 | 19544 | 9772 | 179248178 | 2 | Small businesses | 2 | 20284 | 10142 | 137813202 | 3 | Microenterprises | 2 | 32297 | 16148,5 | 143498740,5 | 4 | Medium-sized enterprises | 2 | 6008 | 3004 | 15635232 | Source: developed by the authors Table 13. Results of one-factor analysis of variance of the "Company size" attribute No. p / p | Source of variation | SS | df | MS | F | P-Value | F critical | 1 | Between groups | 173204721,4 | 3 | 57734907 | 0,484968254 | 0,710748647 | 6,591382116 | 2 | Within groups | 476195352,5 | 4 | 1,19E+08 | | | | 3 | Total | 649400073,9 | 7 | | | | | Source: developed by the authors
Based on the results of the calculation of ANOVA indicators, it can be concluded that this feature "Company size" has absolutely no significance and is not valuable for predicting the execution of contracts, since it has a large P-value equal to 0.71. The data set under study contains about 51 variants of organizational and legal forms of suppliers. The highest frequencies of the attribute values are shown in Table 14. Table 14. Frequency of values of the attribute "Organizational and legal form of the supplier" No. p / p | The value of the attribute "Organizational and legal form of the supplier" | Share in the data set | 1 | Limited liability companies | 74% | 2 | Non-public joint-stock companies | 11% | 3 | Public joint stock companies | 6% | 4 | Municipal unitary enterprises | 4% | 5 | State unitary enterprises of the subjects of the Russian Federation | 2% | 6 | Other | 3% | 7 | total | 100% | Source: developed by the authors Table 15 presents the results of a one-factor analysis of variance of the values of the attribute "Organizational and legal form of the supplier". Table 15. Results of one-factor analysis of variance of the attribute "Organizational and legal form of the supplier" No. p / p | Source of variation | SS | df | MS | F | P-Value | F critical | 1 | Between groups | 5,04E+09 | 50 | 1,01E+08 | 6,749593 | 2,35498E-16 | 1,474801586 | 2 | Within groups | 1,52E+09 | 102 | 14948674 | | | | 3 | Total | 6,57E+09 | 152 | | | | | Source: developed by the authors Based on the results of the calculation of ANOVA indicators, it can be concluded that the potential significance of the attribute "Organizational and legal form of the supplier" for predicting the execution of contracts, since the P-value is extremely small. Consider the "Budget level" attribute (Table 16). Table 16. Interface table for the "Budget level" attribute No. p / p | The value of the "Budget level" attribute | Number of problematic contracts | Number of successful contracts | total | 1 | Budget of the Pension Fund of the Russian Federation | 308 | 731 | 1039 | 2 | Budget of a constituent entity of the Russian Federation | 1662 | 9096 | 10758 | 3 | The budget of the territorial state extra-budgetary fund | 13 | 118 | 131 | 4 | Budget of the Federal Compulsory Health Insurance Fund | 45
| 1142 | 1187 | 5 | Budget of the Social Insurance Fund of the Russian Federation | 307 | 113 | 420 | 6 | Local budget | 1902 | 10565 | 12467 | 7 | Federal budget | 1757 | 6229 | 7986 | 8 | total: | 5994 | 27994 | 33988 | Source: developed by the authors Tables 17 and 18 show its results for the data given above. Table 17. Calculation of averages and variances for groups of the "Budget level" attribute. No. p / p | Groups | Account | The amount | Average | Variance | 1 | Budget of the Pension Fund of the Russian Federation | 2 | 1039 | 519,5 | 89464,5 | 2 | Budget of a constituent entity of the Russian Federation | 2 | 10758 | 5379 | 27632178 | 3 | The budget of the territorial state extra-budgetary fund | 2 | 131 | 65,5 | 5512,5 | 4 | Budget of the Federal Compulsory Health Insurance Fund | 2 | 1187 | 593,5 | 601704,5 | 5 | Budget of the Social Insurance Fund of the Russian Federation | 2 | 420 | 210 | 18818 | 6 | Local budget | 2 | 12467 | 6233,5 | 37523785 | 7 | Federal budget | 2 | 7986 | 3993 | 9999392 | Source: developed by the authors Table 18. Results of one-factor variance analysis of the "Budget level" attribute No. p / p | Source of variation | SS | df | MS | F | P-Value | F critical | 1 | Between groups | 86296297 | 6 | 14382716 | 1,326979 | 0,356718 | 3,865969 | 2 | Within groups | 75870854 | 7 | 10838693 | | | | 3 | Total | 1,62E+08 | 13 | | | |
| Source: developed by the authors Based on the results of the calculation of ANOVA indicators, it can be concluded that this feature is not significant and is not valuable for predicting the execution of contracts, since it has a P-value significantly greater than 0.05. Conclusions Thus, based on the results of the application of the ANOVA method, conclusions were drawn about the potential value for the purposes of forecasting the execution of government contracts of the following features: - "Organizational and legal form of the supplier" - "Supplier's region"; - "The first two characters of the OKVED 2 code"; These signs are not statistically significant: - "Supplier's form of ownership"; - "The size of the supplier company" - "Budget level"; - "Order placement method". The obtained results can be applied by researchers in the course of cluster analysis, research data analysis, in the construction of an ensemble of models for forecasting the execution of government contracts. The practical significance of the study is provided by the complexity and complexity of the task of forecasting government contracts, a large volume of the generated sample. The conclusions do not contradict the research results of other domestic and foreign authors, however, they expand and deepen existing approaches to the search for new significant features based on information capabilities contained in state information systems and other sources of big data. In the future, the authors plan to expand the volume of the data set, continue and deepen the research analysis of data, and build reliable ML models.
References
1. Tikhomirov, P. A. (2020). Register of unscrupulous suppliers: improvement is required. Russian competitive law and economics, 4, 70–83.
2. Yudin, A. A., & Tarabukina, T. V. (2022). Monitoring, audit and control in the contract procurement system. Moscow Economic Journal, 1, 408–418.
3. Styrin, E. M., Rodionova, Yu. D. (2020). Unified information system in the field of procurement as a state digital platform: current state and prospects. Issues of state and municipal management, 3, 49–70.
4. Khosrowshahi, F. (1999). Neural network model for contractors’ prequalification for local authority projects. Engineering, Construction and Architectural Management, 6, 315–328.
5. Eliseev, D. A., Romanov, D. A. (2018). Machine learning: forecasting risks of government procurement. Open Systems. DBMS, 2, 42–44.
6. Acheamfour, V. K., Kissi, E., Adjei-Kumi, T., & Adinyira, E. (2020). Review of empirical arguments on contractor pre-qualification criteria. Journal of Engineering, Design and Technology, 18, 70–83.
7. Sanjana, P., Basavaraj, K., Pranali, D., Kashmira, P. (2020). Review of Contractor Prequalification Criteria and their Impact on Project Success Factors. International Journal of Research in Engineering, Science and Management, 3, 298–302.
8. Sozaeva D.A., Gonchar K.V. (2022). Study of the risks of termination of contracts concluded as a result of public procurement. Problems of risk analysis, 3, 74–85.
9. El-Sawalhi, N., Eaton, D., Rustom, R. (2007). Contractor pre-qualification model: State-of-the-art. International Journal of Project Management, 25, 465–474.
10. Zedan, A. (1996). Contractor selection using multiattrlbute utility theory [DX Reader version]. Retrieved from http://usir.salford.ac.uk/id/eprint/14813/1/DX197696.pdf
11. Tsyganova, M. S., Chernushenko, D. A., Buresh, S. V. (2022). System for forecasting participants in public procurement according to federal laws No. 44-FZ and No. 223-FZ. Bulletin of Cherepovets State University, 3, 61–76.
First Peer Review
Peer reviewers' evaluations remain confidential and are not disclosed to the public. Only external reviews, authorized for publication by the article's author(s), are made public. Typically, these final reviews are conducted after the manuscript's revision. Adhering to our double-blind review policy, the reviewer's identity is kept confidential.
The list of publisher reviewers can be found here.
The subject of the study. The article, based on the title formulated by the author, should be devoted to risk assessment indicators for the execution of government contracts with a long life cycle. The content of the article, in general, does not deviate from the indicated vector, but the issues are disclosed superficially, without detailing and explanations on specific examples. The research methodology is based on the presentation of well-known facts, their partial systematization, analysis and synthesis. It is very valuable that there are graphic objects in the text. This creates a positive impression from reading a scientific article. At the same time, in the diagram shown in Figure 1, you should specify the connections between all the rectangles. When finalizing, the author is also recommended to pay attention to the methods of processing numerical data, which make it possible to clearly demonstrate the application of these indicators in practice. The relevance of the study of issues related to the assessment of the risk of execution of government contracts is beyond doubt, because it meets the tactical and strategic goals of socio-economic development of the Russian Federation. The issues raised are particularly relevant in the context of achieving the goals set in the Decree of the President of Russia dated July 21, 2020. It would be good to show this link in the text: this would significantly expand the potential readership. The scientific novelty in the text presented for review is partially present if these approaches are copyrighted (unfortunately, the source is not indicated under the graphic objects: if borrowed, but it is necessary to specify a link, if copyrighted, then the appropriate signature): For example, in the diagram shown in Figure 1, the author provides a high-level description of the processes of monitoring suppliers and the execution of government contracts. Table 1 is also of interest, but it needs to be improved: it is recommended to divide column 2 into two separate columns: criterion, calculation method. Style, structure, content. From the point of view of the absence of colloquial statements, the style of presentation is scientific. The structure of the article by the author is strictly structured, allows you to deeply reveal the content of the issues under consideration, but the author did not take advantage of this opportunity. From a substantive point of view, the article requires correction, because in fact, algorithms for calculating indicators for monitoring government contracts are stated as the results of the study, but where did they come from? How are they related to the previous text? What is the significance of each of these indicators? What is new in their calculation suggested by the author? It would be interesting for representatives of the potential readership to get answers to these questions from the text of the article. Bibliography. The bibliographic list formed by the author includes 10 sources, mostly published in 2020 or earlier. The author is recommended to expand the list of sources by including relevant publications in 2023 and increasing the number of works in 2022. Moreover, a non-uniform approach to the design of the list of sources attracts attention. It is also recommended that the author add a list of electronic sources, including those containing numerical statistical data. Appeal to opponents. Despite the generated list of sources and quoting individual fragments of them in the text, no discussion was organized at all. When finalizing the article, the author is recommended to discuss the identified problems and developed recommendations for their solution with those that are reflected in the scientific works of other scientists. Conclusions, the interest of the readership. Taking into account all the above, the article requires revision, after which the possibility of its publication may be considered. Taking into account the relevance of the chosen research topic and provided that it is filled with specific numerical examples, substantiated problems and reasoned recommendations for their solution
Second Peer Review
Peer reviewers' evaluations remain confidential and are not disclosed to the public. Only external reviews, authorized for publication by the article's author(s), are made public. Typically, these final reviews are conducted after the manuscript's revision. Adhering to our double-blind review policy, the reviewer's identity is kept confidential.
The list of publisher reviewers can be found here.
The subject of the research in the reviewed article is the risk assessment indicators for the execution of government contracts with a long life cycle, which are considered by the authors in the context of their impact on ensuring state security. The research methodology is based on the processing of statistical data on the execution of government contracts using methods of big data analysis, machine learning and artificial intelligence. The authors rightly attribute the relevance of the work to the fact that the efficiency of the Russian economy is largely determined by the efficiency of its public sector due to the fact that the volume of public procurement is comparable to the revenue side of the federal budget. The scientific novelty of the reviewed study, according to the reviewer, consists in identifying signs of government contracts that may be important for predicting their execution: "Organizational and legal form of the supplier", "Supplier's region", "The first two characters of the OKVED 2 code". The following sections are structurally highlighted in the article: Introduction, Review of existing research, Research Methodology, Research results and discussion, Conclusions and Bibliography. The bibliographic list includes 11 sources – publications of domestic and foreign scientists on the topic of the article, to which there are targeted links in the text confirming the existence of an appeal to opponents. The authors note that within the framework of the current public procurement system in our country, there are many problems in their organization. The article presents an enlarged scheme of the process of regulating the public procurement system in Russia, provides an overview of foreign and domestic literary sources in this area, schematically presents the methodology of the study with the allocation of its main stages; describes the processes of data unloading, preprocessing and enrichment; characterizes the resulting dataset reflecting the number of observations and studied features; provides examples of tables constructed conjugations for the selected categorical features; the expediency of using a one-factor analysis of variance is justified, its results are shown, conclusions are drawn about the features that are important for the success of public procurement and those characteristics that do not significantly affect the execution of contracts. As a comment, it should be noted that the section "Research results and their discussion" contains 13 tables reflecting the results of the work done by the authors, however, much less attention is paid to the discussion and interpretation of the new information and knowledge obtained. And the total number of illustrations – 18 tables and 2 figures - seems superfluous for one journal article, since the meaningful conclusions on them fit into several lines, respectively, a detailed description of the intermediate results clearly prevails over the presentation of the final results of the study. The article reflects the results of the research conducted by the authors, corresponds to the direction of the journal "Security Issues", contains elements of scientific novelty and practical significance, may arouse interest among readers, and is recommended for publication taking into account the comments and wishes expressed.
Link to this article
You can simply select and copy link from below text field.
|
|