|
MAIN PAGE
> Back to contents
Legal Studies
Reference:
Bodrov N.F., Lebedeva A.K.
The concept of deepfake in Russian law, classification of deepfake and issues of their legal regulation
// Legal Studies.
2023. № 11.
P. 26-41.
DOI: 10.25136/2409-7136.2023.11.69014 EDN: DYIHIR URL: https://en.nbpublish.com/library_read_article.php?id=69014
The concept of deepfake in Russian law, classification of deepfake and issues of their legal regulation
Bodrov Nikolay Filippovich
ORCID: 0000-0002-9005-3821
PhD in Law
Associate Professor of the Department of forensic expertise Kutafin Moscow State Law University (MSAL), PhD (Law)
123242, Russia, Moscow region, Moscow, Sadovaya-Kudrinskaya str., 9, room 729
|
bodrovnf@gmail.com
|
|
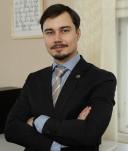 |
|
Lebedeva Antonina Konstantinovna
PhD in Law
Associate professor of Forensic Expertise’s department at Kutafin Moscow State Law University (MSAL)
125993, Russia, Moscow, Sadovaya-Kudrinskaya str., 9
|
tonya109@yandex.ru
|
|
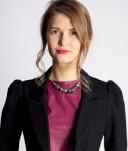 |
|
DOI: 10.25136/2409-7136.2023.11.69014
EDN: DYIHIR
Received:
14-11-2023
Published:
21-11-2023
Abstract:
The article deals with the issues of legal regulation of deepfake in the Russian Federation. Legal regulation of deepfake does not keep up with the pace of development of artificial intelligence technologies. The authors emphasize that there is no definition of deepfake in the current legislation, and the existing formulations in scientific works are extremely contradictory in nature. Taking into account the pace of development of artificial intelligence technologies, it is necessary to legislate the definition of deepfake. The authors note that the classification of deepfakes is fundamentally important for the legal regulation of these technologies. According to the results of the analysis of modern neural networks the species classification of deepfakes is offered. Taking into account the authors' proposed definition of the concept of "deepfake" and taking into account the lack of legal mechanisms to regulate social relations in the sphere of use and distribution of deepfake, which cause the development of digital transformation, it is important to form mechanisms to adapt the legal system to the challenges associated with the development of deepfake technologies.
Keywords:
deepfakes, generative content, legal regulation, deepfake’s classification, neural networks, synthesized speech, criminal law, transformation of law, digital technologies, watermarks
This article is automatically translated.
You can find original text of the article here.
Every day, news about the latest achievements of artificial intelligence appears in the media space. For example, at the presentation of Google "Google I/O", held on May 10, 2023, the abbreviation "AI" (short for "artificial intelligence" (artificial intelligence) was pronounced 146 times. Taking into account the pace of development of artificial intelligence technologies (hereinafter – AI), the availability of products using neural network technologies, there is a need to develop legal mechanisms for regulating the results of the use of AI, and related public relations. The problems of artificial intelligence and related social relations are a fairly broad subject of research, which we will consider from the perspective of criminal law sciences. According to our estimates, the central place in the system of AI performance results is occupied by the so-called deepfake (deepfake), which have universal criminogenic potential. The regulatory and legal regulation of deepfake technologies and materials clearly does not keep pace with the pace of technological development, but in the scientific literature, the problems of threats associated with deepfakes have been discussed since 2019 [See, for example, 3, 7, 10, 12]. The analysis of deepfake technologies and materials, due to the relative novelty of specific knowledge intensity, has now reached a dead end to some extent. Instead of the author's definitions of deepfakes that take into account the specifics of legal regulation, the scientific literature often contains quite primitive information from publicly available sources. This term is used to denote both the AI technology itself and the nomination of fake content itself, which misleads both the scientific community and users. However, the definitions of deepfake, which are given in modern scientific works, do not reveal all the facets of this concept. In formulating a suitable definition of the concept of "deepfake", we analyzed the definitions previously published in the scientific literature because, in our opinion, they do not fully reflect the essence of this concept. For example, definitions are common in scientific papers, the authors of which cite the concept of a deepfake without taking into account the possibility of creating content in the form of sound: "Deepfakes" are synthetically produced media content in which the original person (the one who is originally in the image) is replaced by another person" [6, p. 117]. It should also be noted that a person is not always "replaced by another person", there is a possibility of replacing not only the image of the face or head, but also the transformation of articulation and facial expressions. In addition, deepfake may not be related to the generation of a person's image at all, we can also talk about the generation, for example, of a specific place. It seems to us that the approach to the analysis of deepfakes is too narrow, when attention is focused on the deliberate distortion of objects. For example, in the definition: "Deepfakes are intentionally distorted audio, video or other files using deep learning technology (the definition is derived from the phrase "deep learning" - deep learning, "fake" - fake), which depict something fictitious or false, which allows attackers to use a new and complex tool of social engineering" [4, P. 74] the author points out the deliberate nature of the distortion of audio, video or other files. However, deepfake is not some kind of program for post–processing files in order to change them, like, for example, voicechanger (a class of software products for changing the voice), which either through post-processing or in real time change the speaker's voice according to an algorithm pre-programmed in the program. A deepfake is created by generating a digital product based on neural network training on a dataset. It can be huge amounts of Internet data, as well as, for example, phonograms with a recording of the sounding speech of a particular person. The "dataset" in accordance with Article 5 of the "National Strategy for the Development of Artificial Intelligence for the period up to 2030", approved by the Decree of the President of the Russian Federation dated 10.10.2019 N 490 "On the development of artificial intelligence in the Russian Federation" should be understood as: "e) a data set is a set of data that has undergone preliminary preparation (processing) in accordance with the requirements of the legislation of the Russian Federation on information, information technologies and information protection and is necessary for the development of software based on artificial intelligence." Taking into account the analyzed specifics, it is necessary to approach the definition of a deepfake, first of all, as a digital product. Deepfake in its modern view is the result (product) generated using neural network technologies. It is also possible to imagine an analog form of representation of a deepfake, for example, a material published in periodicals containing an image or text, fully or partially generated using neural network technologies. But the primary source of such information will certainly be a digital product – the result of synthesis using neural network technologies. A deepfake in digital form can be represented by different types of content or their combinations. Therefore, in order to formulate the definition of the concept of "Deepfake", it is important, in our opinion, to determine in what form the analyzed digital product can be presented. It seems important to us to analyze the types of deepfakes by the types of generated content or their combinations. 1. Graphics.
Modern technologies allow the synthesis of graphics in the form of individual images or video phonograms. 1.1. Images. Over the past six months, several dozen neural networks have appeared in the Internet space, most of which use the text query interface (prompt) for image synthesis. Text-to-image (image generation by text query) models allow you to generate a variety of images, including synthesis based on reference images. The popular neural network "Midjourney" generates images with the ability to create a graphic of a photograph (indicating the camera model and the optics used), as close as possible to the real image, if the user's task (prompt) contained a request for it (for example, "hyperrealistic"). Even for short queries, this neural network demonstrates results that can mislead the layman. The user can set the image quality, from which device it was "taken", the location of the lens, the focal length and other parameters of real photography. In addition, there is a command to generate an image from a photo. For example, a user can upload a photo of the desired face and generate almost any situation with it. Of course, the administration of the neural network has developed some principles that restrict the "freedom of creativity". For example, "Do not create images and do not use text prompts that are inherently disrespectful, aggressive or offensive. Violence or harassment of any kind is unacceptable; No adult content or blood. Please avoid visually shocking or disturbing content. We will automatically block the input of some texts" [21]. The "Midjourney" neural network access service (on the Discord platform) allows users to perform administration functions (a kind of social control by users) of the results of generation by filing complaints. However, in order to create a misleading digital product, it is not always necessary to create a graphic image of scenes of violence, cruelty, pornography, etc. For example, to manipulate the electorate in elections, it may be enough to generate a joint photo of a representative of the leading party and, let's say, some war criminal sitting at the negotiating table. In addition to the mentioned neural network, there are, for example, the following: Crayon (formerly Dall-E mini). The neural network is from Microsoft developers, who emphasize that the neural network creates extremely realistic images, that it even makes them worry that the generation of such images can lead to unpredictable consequences when creating deepfakes. Kandinsky 2.2 is a neural network from the developers of Sber with the partner support of scientists from the AI Institute of Artificial Intelligence on the combined data set of Sber AI and SberDevices. In addition to generating images from text, this neural network can finish drawing uploaded photos, as well as modify them, for example, redraw an existing image in a different style or combine two images. Masterpiece. The neural network from Yandex developers also has its own mobile application, according to existing information, the neural network was trained on 240 million examples, which were images with text descriptions. To improve the quality, neural network training continues. In May 2023, Adobe Photoshop introduced a new Generative Fill tool, based on AI, this tool allows you to add, expand or delete the contents of images without making irreversible changes using simple text queries. The generative fill functionality includes, for example, the function of removing objects from a photo, when the space instead of an object (a person, a car trace) is automatically replaced by a background (without the usual traces of processing with the tools of a graphic editor). A characteristic feature of the technological limitations of generative fill at the present stage is the resolution of 1024 x 1024 (1MP), but the experience of analyzing images attached to case materials shows that such fill capabilities are more than sufficient for the average graphics used in judicial evidence. 1.2. Video recordings. Speaking of deepfakes, modern Internet users usually present video phonograms in the first place. Examples of programs can easily be found on the Internet. One of the most famous is DeepFaceLab. With this program, you can replace the face in the video, change the age of the speaker on the video, change not just the face, but the shape of the head, hairstyle, voice on the soundtrack completely, and if you have average video editing skills (for example, in Adobe After Effects or Davinci Resolve), you can transform the articulation and facial expressions on the video in addition to a system for cloning sounding speech. "DeepFaceLive" - allows you to replace the face both on the video, and in the process of online communication or in the process of streaming on the webcam. "Face 2 Face" - puts the mimicry of the managing "actor" on any other face. "Zao Deepfake" is a Chinese application that works on the basis of trained neural networks, allows you to replace not just one face photo with another, but all facial expressions and facial movements in a video.
This list can be continued further, but for a general idea of the functionality of such programs, it is enough to describe the examples we have mentioned. 2. Sound. If in September 2019 it was said that "imitation" of a person's voice is an extremely time-consuming and difficult process: "It is expensive and inefficient to train artificial intelligence to imitate the voice of a certain person, says Artem Sychev, deputy head of the Information Security Department of the Central Bank: "The applicability of such fraud methods is extremely low. To do this, the attacker must know for sure that the victim will react correctly to this voice" [9]. Now some services offer to clone your speech, learning on small phonograms and offering extremely high quality audio signal without any significant requirements for the user and the equipment available to him. Sounding speech synthesis is a technology that allows you to convert text into sounding speech (TTS-speech synthesis technologies (Text-to-Speech) [see, for example, 1, 2, 5]. Speaking about the synthesis of sounding speech from the text, we need to separate it from the concept of "speech cloning" (from the English. voice cloning). Analyzing the synthesis technologies of sounding speech, it is important to note that it is about voicing content with the voice of a "simulated" speaker with the specified parameters. Thanks to deep learning technology, a neural network can be trained on phonograms with the voice and speech of millions of speakers. However, when the neural network is faced with the task of generating the sounding speech of a particular person, the neural network is trained on phonograms with samples of voice and speech of an individually defined speaker. This type of synthesis of sounding speech is commonly called "cloning of sounding speech", thus, artificially, using AI technologies, the sounding speech of a real person is generated. From a legal point of view, it is important to take into account the fact that the technology of generating sounding speech is at the junction with such an important industry as biometric voice identification. Biometric identification/authorization by voice is already being used as an independent tool or component of information systems in the banking sector [11]. For example, in JSC "Tinkoff Bank" in October 2014, a similar technology was introduced [24]. In fact, identification/authentication and cloning of sounding speech are competing technologies. Legal regulation of legal relations in such an area should pursue not only the task of keeping up with the level of technological development, but also take into account the risks of lack of adequate and detailed regulation in the aspect of information security. Let's consider some systems of sounding speech synthesis and their capabilities. VALL-E allows you to synthesize personalized high-quality speech. Based on the developed algorithms and the already implemented neural network training, VALL-E can clone the voice and speech of a particular speaker, using, according to the developers, 3-second recordings, while preserving the emotions of the speaker and the acoustic environment, if it was in the user's request. This neural network also allows you to create text content using generative AI models, for example, such as GPT-3 [16]. In May 2023, a publication was published with a description and examples of the generation of sounding speech "SoundStorm" [27]. This model demonstrates high results of speech cloning. The authors of the publication, as follows from the materials published by them, are aware of the possibilities of using their model for, for example, bypassing biometric identification systems. The authors point out that, taking into account the possible use of the model for illegal purposes, in the future they plan to study approaches to the detection of synthesized speech, for example, sound watermarks, so that any potential use of this technology strictly complies with the responsible AI principles that the creators of the model adhere to. ElevenLabs. As the developers point out, they represent the most realistic software for converting text into sounding speech [17]. The fakeyou service [19] provides users with the opportunity to clone the voice of any person, before subscribing, it is necessary to answer several questions for what purposes it is planned to use the voice whose voice will be cloned. To train a neural network, you can either record phonograms through the service itself, or attach your own high-quality recordings. There are also services that initially do not set the main goal of speech synthesis and cloning, but offer it to users. For example, Descript, this service was created initially for the convenience of the creators of podcasts and videos, as well as their processing. Here are some of its functions: – an editor that simultaneously allows you to process and modify audio, text and video, – the program performs speech recognition and provides a "transcript" with the text of its verbatim content, – when editing the received text, changes also occur in the audio material,
– when words are deleted, the program automatically deletes the relevant fragments of the video. However, the developers also offer technologies for high-quality synthesis and cloning of sounding speech. Lyrebird is the AI research department at Descript, creating a new generation of tools for editing and synthesizing media content. Using the capabilities of artificial intelligence, Descript generates phrases, accurately imitating the features of a person's voice. The program can voice the entered text both with voices from the existing collection and with the user's own voice. Russian Russian is not supported by the program, it does not recognize or synthesize Russian speech. The ethics statement of this company states that after training speech models on your voice samples (sound recording begins only after recording the user's oral consent file), the user, as the owner of the "digital voice", after generation controls when and for what purposes the synthesis results are used. Thus, here and in the other examples we have mentioned, the industry's request for the creation of industry standards to combat disinformation is clearly traced. 3. Text The greatest rates of improvement at the present stage of the development of artificial intelligence systems are demonstrated by systems based on the use of large language models (BYAM) [18]. Due to the significant successes of the architecture of the so-called trans-dimensional [14] deep neural networks, BYAM by the type of generative pre-trained transformers (GPT) have become widespread. The most popularized among such neural networks is ChatGPT [15]. The current version, GPT-4, is a language model developed by OpenAI, based on the transformer architecture, or rather, a variant of the transformer model known as a transformer decoder. At its core, ChatGPT is a machine learning model designed to generate a "human-like" text, that is, an attempt to synthesize written speech that meets the criteria of logic, meaningfulness, grammatical correctness, etc. The neural network was trained by machine learning, known as "learning without observation". With this approach, the model is provided with a large corpus of text data, and it learns to predict the next word in a sentence. In particular, GPT (Generative Pretrained Transformer) models are trained using a variant of unsupervised learning called self-supervised or self-supervised learning. GPT-4, like its predecessors, but on a larger scale, uses a huge amount of data and computing resources for training, operating in its work, according to various estimates, about 175 billion parameters (taking into account the fact that accurate data, as for most proprietary neural networks, is not publicly available). Taking into account the problems we are considering, large language models are of practical interest in terms of the possibility of generating texts written or intended for voicing speech on behalf of a particular person. Already, users have the opportunity to set such parameters to the neural network so that it generates the text of the desired topic on samples of publicly available written speech. The samples can be messages from messengers, e-mail correspondence, public records of social networks, etc. It is possible to formulate a task (prompt) and compose a text on a given topic in the style of the author (specify the style parameters: the dictionary (lexical stock) of the author, the peculiarities of the use of syntactic constructions, the manner of speech, etc.). The study of such queries is already being carried out in an independent direction of applied research – prompt engineering (tracing paper from the English prompt engineering), one of the main tasks of which is the selection and structuring of modifications of the text of queries (promptov) to solve specific tasks for example, generating text on behalf of a given author. Today there is a technical possibility of generating texts on any subject. It is characteristic that there are already some mechanisms of ethical self-regulation of neural network software products. The Open AI Corporation, which is the creators of the neural network, indicate that the user's safety is in the first place, however, there are instructions on how to "deceive the neural network" and make prompta that allow you to bypass the specified moral and ethical principles, as well as generate text without the principle of user security. Among the ChatGPT analogues in the public domain is, for example, Bard AI, an experimental project from Google, which, like ChatGPT in the form of a chatbot, conducts a conversation with the user and can generate a variety of texts on requests. An important aspect of the problem under consideration is the accessibility of text synthesis technologies. For example, ChatGPT neural network resources are now available to virtually every user of the Bing search engine [20], distributed by Microsoft Corporation as a pre-installed browser program. Taking into account the general availability, it is possible to draw some analogies with intellectual property law, which is faced with the need for legal regulation of public relations in conditions of virtually unlimited access to information. The problems of deepfake technologies, among other things, are also characterized by an extremely high degree of public danger, which, according to our estimates, will become one of the primary factors of information security in the near future.
4. Sound+Graphics Combination A combination of several forms of representation, for example, in the form of a combination of generated sound and graphics, is already found in the form of a so-called "digital avatar". A number of scientific works in the legal literature have already been devoted to the legal understanding of this category [see, for example, 8]. Already several services offer users to create their own digital avatar. For example, the service "Synthesia" [23] offers users to create their own "AI avatar", that is, an avatar created using artificial intelligence technologies. As the developers point out, their service can create a photorealistic image of a real person, as well as clone his voice. A digital avatar is created on the basis of 15-minute videos of the user, and voice cloning is carried out on the basis of training the system on reading a reference text prepared in advance by the service. With the help of this service, journalist Joana Stern created her own digital avatar, which [26] was used by her to overcome the mechanism of the biometric verification system by the voice of the bank, as well as to mislead the relatives of the journalist. And when using the chat bot ChatGPT, it is possible to train a neural network on samples of written or oral speech of a particular person and synthesize not only her voice and appearance, but also the content side of speech. Thus, this form of deepfake can go into the following: a combination of text, graphics, sound. In practice, cases of aggregation of various types of content generated using neural networks are already known. For example, in June 2023, a church service was held in a Protestant church in Germany, almost completely generated using AI technologies [13]. The 40-minute service, including a sermon, prayers and music, was created using ChatGPT, and the service was "managed" by four different digital avatars on the screen, two young women and two young men. The DeepFaceLab system we mentioned earlier allows, if you have some skills in video editing (for example, Adobe After Effects or Davinci Resolve programs), to change the movement of the lips of the face on the video. When using the cloning systems of sounding speech on the example presented by the developers, this neural network allowed the creator of the deepfake to articulate the necessary phrases using the appearance of famous political figures. 5. A combination of text, graphics, and sound. From a commercial point of view, the combination of text, graphics, and sound in one material is even more popular type of content, since its development is closely related to the requests of various kinds of corporate industry (for example, the creation of automated news broadcasting services, reference services, etc.). Despite the high-tech nature of this kind of content, at the present stage its generation is increasingly available to the layman. For example, the Chinese company Tencent Cloud announced the launch of a digital platform for creating people - deepfakes-as-a-Service (DFaaS) [25]. According to the developers, for a relatively small fee, the service allows you to create high-definition digital copies of everyone, using only three minutes of live video and 100 spoken phrases. It will take about 24 hours after the samples are entered to complete the digital avatar generation process. That is, technological capabilities allow for such generation without requiring the user to attract their own computing resources, besides allowing you to get the result in a short time. For example, the creators of the service "Spiritme" [22] offer users the rapid creation of videos with digital avatars. To create a digital avatar, the user needs to record a five-minute video of his own appearance, and oral speech on any topic can be used as a sample. Then the service generates a digital avatar of the user, which can pronounce any text that you prepare, with a sufficient degree of realism, as well as displaying the user's appearance, features of his voice and emotions. Taking into account the above, it is extremely difficult to overestimate the criminogenic potential of this kind of content, especially taking into account the degree of awareness of the average user and in conditions of a shortage of legal regulation of technologies and synthesis results in the domestic legal system. Analyzing the practice in cases related to the illegal distribution of deepfake content and potential threats emanating from deepfakes, two main purposes of their use can be formulated: misleading or overcoming access control and management systems by the user. The results of the analysis of the essence of deepfake content allow us to formulate the following author's definition of deepfakes. Deepfake is a digital product in the form of text, graphics, sound or a combination of them, generated in whole or in part using neural network technologies, for the purpose of misleading or overcoming access control and management systems by the user. In the context of the definition of the concept of "deepfake" and taking into account the lack of legal mechanisms for regulating public relations, as well as at the stage of formation of a number of end-to-end digital technologies that determine the development of digital transformation, it is important to form mechanisms for adapting the legal system to the challenges associated with the development of deepfake technologies. The following measures seem to us to be of primary importance in matters of legal regulation of such technologies and the results of their application:
- normative consolidation of the term deepfake and its definition in domestic legislation, taking into account technological capabilities and risks caused by the development and widespread spread of such technologies. - making additions to the norms of criminal legislation and legislation on administrative offenses related to the use of deepfake content as the subject of illegal (including criminal) encroachment, an element of the method of committing an offense (including a crime), circumstances aggravating liability, since deepfake is a more sophisticated high-tech product that has fundamentally a high level of public danger and difficult to recognize at the current level of development of forensic technology. - making additions to the norms of civil legislation, for example, in terms of protecting honor, dignity and business reputation, as well as protecting the image of a citizen, since Articles 152 and 152.1 of the Civil Code of the Russian Federation do not adequately reflect the realities of illegal distribution of fake content that have already become modern. - an obvious step in the regulation of artificial intelligence and related public relations is the development of norms of relevant legislation on artificial intelligence systems, robotics, virtual reality, big data. In the public legal sphere, first of all, it is necessary to settle issues related to the responsibility of developers of neural network technologies, since the subject of legal regulation primarily depends on the trajectory of their development. An effective tool for controlling the spread of deepfakes can be the inclusion in the body of files generated by neural networks of watermarks (from the English watermark) and additional service information in metadata. In summary, it is worth noting that deepfake technologies in the context of modern digital transformation are poorly studied, but one of the most intensively developing areas with high criminogenic potential. We will have to fully assess the risks associated with the development of this sphere in the very near future, and the volume and nature of illegal activity in this rapidly developing sphere directly depends on the quality of regulatory and legal regulation of the scope of artificial intelligence.
References
1. Bodrov, N. F., & Lebedeva, A. K. (2021). Prospects of forensic investigation of synthesized sounding speech. Laws of Russia: experience, analysis, practice, 3, 9-13.
2. Bodrov, N. F., & Lebedeva, A. K. (2021). Forensic research of synthesized sounding speech. Socio-economic development and the quality of the legal environment : Collection of reports VIII Moscow Legal Forum (XIX International Scientific and Practical Conference): in 5 parts, Moscow. Moscow State Law University (MSAL), 4, 263-266.
3. Danilenko, Y. A. (2019). Problems of investigation of certain types of cybercrimes committed with the use of artificial intelligence. Problems of obtaining and using evidentiary and criminally significant information, 37-39.
4. Ignatenkov, G. K. (2022). Deepfake technology as a threat to information security. Nauka. Research. Practice : Collection of selected articles on the materials of the International Scientific Conference, St. Petersburg, June 25, 2022. St. Petersburg: Private scientific and educational institution of additional professional education Humanitarian National Research Institute "NACRAZVITIE", 74-77.
5. Lebedeva, A. K. (2020). Technologies of voice synthesis and forensic phonoscopic examination. Vestnik kriminalistiki, 3(75), 55-60.
6. Luzhinskaya, E. L. (2022). Peculiarities of the study of images of human appearance, changed by means of software. Questions of criminalistics, criminology, and forensic examination, 2(52), 116-121.
7. Ovchinnikov, A.V. (2022). Distribution of Deepfakes in the Internet Space: Problematic aspects of legal regulation. Problems of improvement of the Russian legislation: Collection of abstracts of the All-Russian (with international participation) scientific conference of cadets, listeners and students, Barnaul, 245-255.
8. Rybakov, O. Y. (2020). Man, law, digital technologies: modern directions of research (review of the All-Russian scientific and practical online conference). Monitoring of law enforcement, 2(35), 83-87.
9. Network edition "Kommersant". (2023). Retrieved from https://www.kommersant.ru/doc/4081979.
10. Smirnov, A. A. (2019). "Deep Fakes". Essence and assessment of potential impact on national security. Free Thought, 5(1677), 63-84.
11. Remote identification | Bank of Russia.(2023). Retrieved from https://www.cbr.ru/fintech/digital_biometric_id/
12. Yavorsky, M. A., & Mavrinskaya T. V. (2019). Deepfake: legal problems and their solution. Actual problems of legal system development in the digital era, 134-138.
13. Apnews. (2023). Retrieved from https://apnews.com/article/germany-church-protestants-chatgpt-ai-sermon-651f21c24cfb47e3122e987a7263d348
14. Attention is All you Need. (2017). A. Vaswani. Advances in Neural Information Processing Systems. I. Guyon. (Т. 30., pp.1-15). Curran Associates, Inc.
15. OpenAI. (2023). ChatGPT (version September 25) [large language model ]. Retrieved from https://chat.openai.com
16. Chengyi, Wang, Sanyuan, Chen, Yu Wu, Ziqiang, Zhang Long, Zhou, Shujie Liu, Zhuo, Chen, Yanqing, Liu Huaming Wang, Jinyu, Li, Lei He Sheng, Zhao, Furu Wei (Microsoft).(2023) Neural Codec Language Models are Zero-Shot Text to Speech Synthesizers. Retrieved from https://lifeiteng.github.io/valle/index.html
17. ElevenLabs. (2023). Retrieved from https://beta.elevenlabs.io/speech-synthesis
18. Carlini, N. Extracting Training Data from Large Language Models. (2020). USENIX Security Symposium. (pp. 1-19).
19. Fakeyou. (2023). Retrieved from https://fakeyou.com/clone
20. Mehdi, Y. Reinventing search with a new AI-powered Microsoft Bing and Edge, your copilot for the web. (2023). Retrieved from https://blogs.microsoft.com/blog/2023/02/07/reinventing-search-with-a-new-ai-powered-microsoft-bing-and-edge-your-copilot-for-the-web/
21. Midjourney.su. (2023). Retrieved from: https://midjourney.su/article/usloviya-servisa/?ysclid=libsesc661284902146
22. SpiritMe. (2023). Retrieved from https://spiritme.tech
23. Synthesia. (2023). Retrieved from https://www.synthesia.io/
24. TCS Bank Is First Among Russian Banks to Introduce Voice‑Authentication System for Its Call Centre – Tinkoff news. (2023). Retrieved from https://www.tinkoff.ru/about/news/21102014-tcs-introduce-voice-authentication-system/
25. Tencent Cloud. (2023). Retrieved from https://www.tencentcloud.com/
26. The Wall Street Journal. (2023). Retrieved from https://www.wsj.com/articles/i-cloned-myself-with-ai-she-fooled-my-bank-and-my-family-356bd1a3
27. Borsos, Zalán, Sharifi, Matt, Vincent, Damien, Kharitonov, Eugene, Zeghidour, Neil, Tagliasacchi, Marco. SoundStorm: Efficient Parallel Audio Generation. (2023). Retrieved from https://google-research.github.io/seanet/soundstorm/examples/
Peer Review
Peer reviewers' evaluations remain confidential and are not disclosed to the public. Only external reviews, authorized for publication by the article's author(s), are made public. Typically, these final reviews are conducted after the manuscript's revision. Adhering to our double-blind review policy, the reviewer's identity is kept confidential.
The list of publisher reviewers can be found here.
The subject of the research in the article submitted for review is, as follows from its name, the concept of a deepfake in Russian law, the classification of deepfakes and issues of their legal regulation. The stated boundaries of the study are indicated by the author ("The problems of artificial intelligence and related public relations represent a fairly broad subject of research, which we will consider from the perspective of criminal law sciences"), but in fact the content of the article goes beyond the narrow "criminal law" framework and corresponds to its "industry-wide" name. However, the title of the work is somewhat cumbersome and is more suitable for academic rather than scientific work. Therefore, it is recommended to change it to "The phenomenon of deepfakes: topical issues of legal regulation", etc.). The methodology of the research is not disclosed in the text of the article, but it is obvious that scientists used universal dialectical, logical, statistical, formal legal, comparative legal research methods. The relevance of the research topic chosen by the author is undeniable and justified by him as follows: "Every day news about the latest achievements of artificial intelligence appears in the media space. For example, at the presentation of Google "Google I/O", held on May 10, 2023, the abbreviation "AI" (short for "artificial intelligence" (artificial intelligence) was pronounced 146 times. Taking into account the pace of development of artificial intelligence technologies (hereinafter – AI), the availability of products using neural network technologies, there is a need to develop legal mechanisms for regulating the results of the use of AI, and related public relations"; "According to our estimates, the so-called deepfake occupies a central place in the AI performance system. possessing universal criminogenic potential." The scientists revealed the degree of study of the problems considered in the article: "The regulatory and legal regulation of deepfake technologies and materials clearly does not keep pace with the pace of technological development, but in the scientific literature the problem of threats associated with deepfakes has been discussed since 2019 [See, for example, 3, 7, 10, 12]. Due to the relative novelty of the specific science intensity, the analysis of deepfake technologies and materials has now reached a dead end to some extent. Instead of the author's definitions of deepfakes, taking into account the specifics of legal regulation, the scientific literature often contains rather primitive information from publicly available sources." The scientific novelty of the work is manifested in the formation by the author of a modern holistic view of the deepfake phenomenon and its types. A number of conclusions of the scientist are of immediate interest: "... the criminogenic potential of this kind of content is extremely difficult to overestimate, especially given the degree of awareness of the average user and in conditions of a shortage of legal regulation of technologies and synthesis results in the domestic legal system. Analyzing the practice in cases related to the illegal distribution of deepfake content and the potential threats posed by deepfakes, two main purposes of their use can be formulated: misleading or overcoming access control and management systems by the user. The results of the analysis of the essence of deepfake content allow us to formulate the following author's definition of deepfakes. Deepfake is a digital product in the form of text, graphics, sound or a combination of them, generated in whole or in part using neural network technologies, for the purpose of misleading or overcoming access control and management systems by the user." The author makes a number of valuable proposals from a theoretical and practical point of view: "... the normative consolidation of the term deepfake and its definition in domestic legislation, taking into account the technological capabilities and risks caused by the development and widespread dissemination of such technologies; amendments to the norms of criminal law and legislation on administrative offenses related to the use of deepfake content as a subject illegal (including criminal) encroachment, an element of the method of committing an offense (including a crime), an aggravating circumstance, since deepfake is a more sophisticated high-tech product with a fundamentally high level of public danger and difficult to recognize at the current level of development of forensic technology; making additions to the norms of civil legislation, for example, in terms of protecting honor, dignity and business reputation, as well as protecting the image of a citizen, since Articles 152 and 152.1 of the Civil Code of the Russian Federation do not adequately reflect the realities of illegal distribution of fake content that have already become modern; an obvious step in regulating artificial intelligence and related public relations is the development of norms of relevant legislation on artificial intelligence systems, robotics, virtual reality, big data." Thus, the article makes a certain contribution to the development of domestic legal science and, of course, deserves the attention of the readership. The scientific style of the research is fully sustained by the author. The structure of the work is quite logical. In the introductory part of the article, the scientist substantiates the relevance of his chosen research topic. In the main part of the work, the author carries out a critical analysis of the main theoretical approaches to understanding the essence of the concept of "deepfake", examines the types of deepfakes by types of generated content or their combinations, offers an original definition of the concept under study, as well as a set of measures to improve the relevant legal regulation. The final part of the article contains conclusions based on the results of the study. The content of the article fully corresponds to its title and does not cause any special complaints. The bibliography of the study is presented by 27 sources (scientific articles, analytical and journalistic materials), including in English. From a formal and factual point of view, this is quite enough. The nature and number of sources used in writing the article allowed the author to reveal the research topic with the necessary depth and completeness. There is an appeal to opponents, both general and private (E. L. Luzhinskaya, G. K. Ignatenkov, etc.), and it is quite sufficient. the scientific discussion is conducted by the author correctly. The provisions of the work are justified to the necessary extent.
Conclusions based on the results of the study are available ("Analyzing the practice in cases related to the illegal distribution of deepfake content and potential threats posed by deepfakes, two main goals of their use can be formulated: misleading or overcoming access control and management systems by the user ..... Deepfake is a digital product in the form of text graphics, sound, or a combination of them, generated in whole or in part using neural network technologies, in order to mislead or overcome access control and management systems by the user. ... The following measures seem to be of primary importance in matters of legal regulation of such technologies and the results of their application: - normative consolidation of the term deepfake and its definition in domestic legislation, taking into account technological capabilities and risks caused by the development and widespread dissemination of such technologies. - making additions to the norms of criminal law and legislation on administrative offenses related to the use of deepfake content as the subject of illegal (including criminal) encroachment, an element of the method of committing an offense (including a crime), circumstances aggravating liability, since deepfake is a more sophisticated high-tech product that has fundamentally a high level of public danger and difficult to recognize at the current level of development of forensic technology. - making additions to the norms of civil legislation, for example, in terms of protecting honor, dignity and business reputation, as well as protecting the image of a citizen, since Articles 152 and 152.1 of the Civil Code of the Russian Federation do not adequately reflect the realities of illegal distribution of fake content that have already become modern. - an obvious step in the regulation of artificial intelligence and related public relations is the development of norms of relevant legislation on artificial intelligence systems, robotics, virtual reality, and big data. In the public legal sphere, first of all, it is necessary to resolve issues related to the responsibility of developers of neural network technologies, since the subject of legal regulation primarily depends on the trajectory of their development. An effective tool for controlling the spread of deepfakes can be the inclusion in the body of files generated by neural networks of watermarks (from the English watermark) and additional service information in metadata"), they are clear, specific, have the properties of scientific novelty, reliability and validity. Thus, the conclusions deserve the attention of the readership. There are typos in the article, so it needs additional proofreading. So, the author writes: "For example, at the presentation of Google "Google I/O", held on May 10, 2023, the abbreviation "AI" (short for "artificial intelligence" (artificial intelligence) was pronounced 146 times" - "it was pronounced". The scientist notes: "Now some services offer to clone your speech, learning from small phonograms and offering extremely high-quality audio signal without any significant requirements for the user and the equipment available to him" - a typo at the beginning of the sentence. The author indicates: "From a legal point of view, it is important to take into account the fact that the technology of sounding speech generation is at the junction with such an important industry as biometric voice identification" - "generation technology", and not "generation technology". The interest of the readership in the article submitted for review can be shown primarily by specialists in the field of theory of state and law, information law, civil law, administrative law, criminal law, provided that it is slightly improved: clarifying the title of the work, disclosing the research methodology, eliminating violations in the design of the article (typos).
Link to this article
You can simply select and copy link from below text field.
|
|